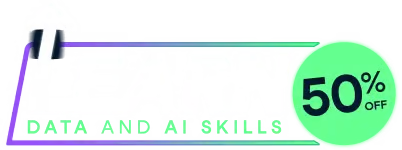
Last chance! 50% off unlimited learning
Sale ends in
Spherical regression using the SESPC distribution.
sespc.reg(y, x, con = TRUE, xnew = NULL, lati = 10, longi = 10, tol = 1e-06)
A list including:
The log-likelihood of the regression model.
A vector with three numbers. A measure of fit of the estimated values, defined as
The two
The beta coefficients.
The standard error of the beta coefficients.
The fitted values of xnew if "xnew" is NULL. If it is not NULL, the fitted values for the "xnew" you supplied will be returned.
A matrix with 3 columns containing the (unit vector) spherical data.
The predictor variable(s), they can be continnuous, spherical, categorical or a mix of them.
Do you want the constant term in the regression?
If you have new data use it, otherwise leave it NULL.
A positive number determing the range of degrees to move left and right from the latitude center. This number and the next determine the grid of points to search for the Q matrix described in Tsagris and Alzeley (2023).
A positive number determing the range of degrees to move up and down from the longitude center. This number and the previous determine the grid of points to search for the Q matrix described in Tsagris and Alzeley (2023).
A tolerance value to decide when to stop the successive optimizations.
Michail Tsagris.
R implementation and documentation: Michail Tsagris mtsagris@uoc.gr.
Regression based on the SESPC distribution (Tsagris and Alzeley, 2023) is applied.
Tsagris M. and Alzeley O. (2023). Circular and spherical projected Cauchy distributions: A Novel Framework for Circular and Directional Data Modeling. https://arxiv.org/pdf/2302.02468.pdf
esag.mle, iag.reg, spml.reg
y <- rsespc( 150, rnorm(3), c(1, 1) )
## this is a small example to pass CRAN's check because the default argument values
## of lati and longi require many seconds
a <- sespc.reg(y, iris[, 4], lati = 2, longi = 2)
Run the code above in your browser using DataLab